Wikipedia
Our Approach to Building Energy Performance
Global Energy Use & Emissions
Currently, a large portion of global energy demand is associated with the built environment, and a considerable segment of the total CO2 emissions comes from fossil fuel consumption in buildings. Of approximately 38% of total GHG emissions related to the building industry, 28% derives from building operation and 10% from the materials used in buildings' construction and maintenance.
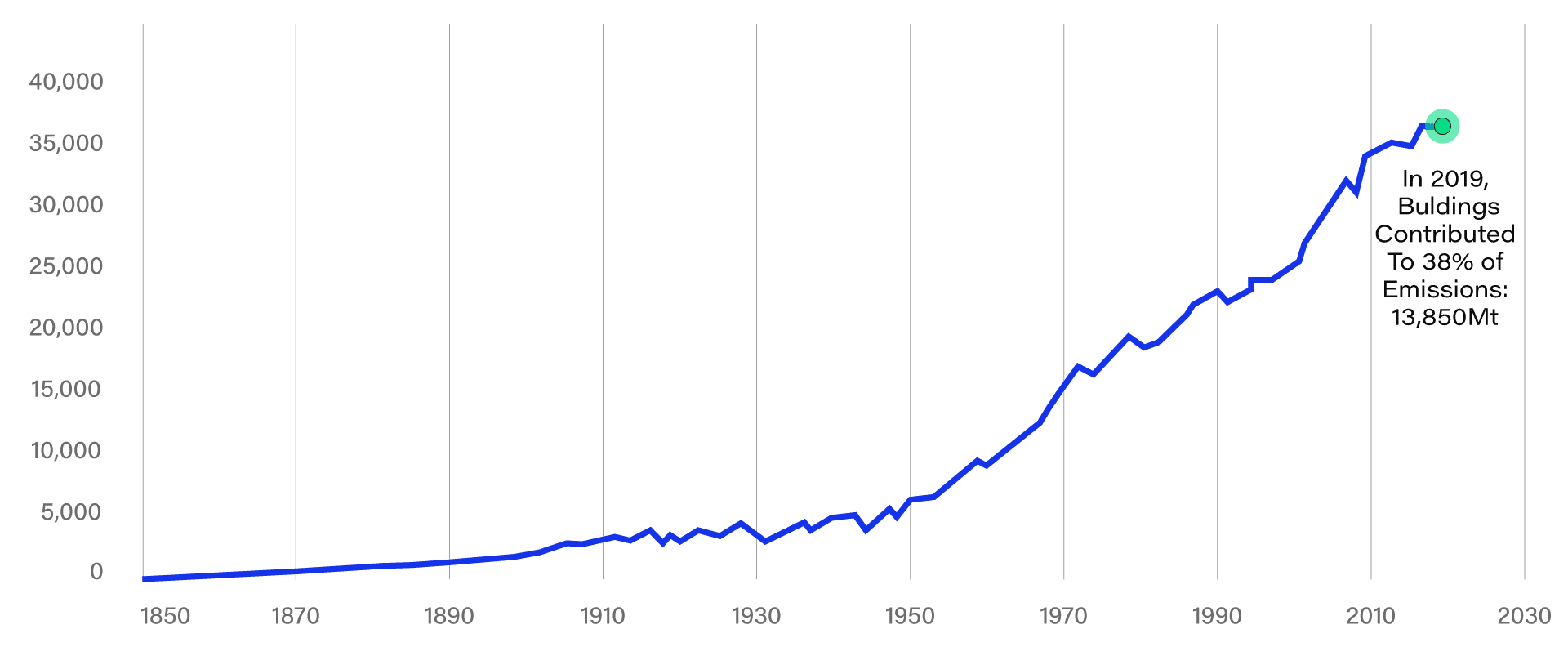
Global annual CO2 emissions (Mt), Our World in Data and Global ABC/IEA/UNEP (2020)
A combination of prescriptive and performance codes, initiatives, and plans have been set by countries, states, and cities around the globe to drive the reduction of energy consumption and CO2 emissions and promote the development of zero net energy buildings.
Building Energy Modeling & Simulation
The architecture, engineering, and construction (AEC) community have taken a great deal of action to reduce energy consumption. Various analytical techniques have been developed to predict and explore buildings’ energy performance. Computational modeling and simulation are among the most powerful and widely used analytical techniques.
- Computational modeling refers to creating a computer-based representation of a real system at a particular level of abstraction
- Computational simulation refers to enacting, implementing, or instantiating a model to predict the future behavior of a real system
Nevertheless, it is very important to recognize that simulation does not directly generate solutions or answers – its main purpose is to increase understanding of the relationship between building parameters and energy performance. Energy modeling and simulation tools, ranging from reduced-order models to highly detailed dynamic calculations, are traditionally used by engineers when the building is formed. Although it is critical to apply energy simulation from the early stage of building design (known as the conceptual stage), the extensive sets of technical input that might not be available at the early stages and the cumbersome process of modeling the building (while it’s changing continuously) make the application of simulation difficult for architects. Building designers and architects are still looking for appropriate tools and frameworks to help them explore all design possibilities and make energy-informed decisions from the concept development stage.
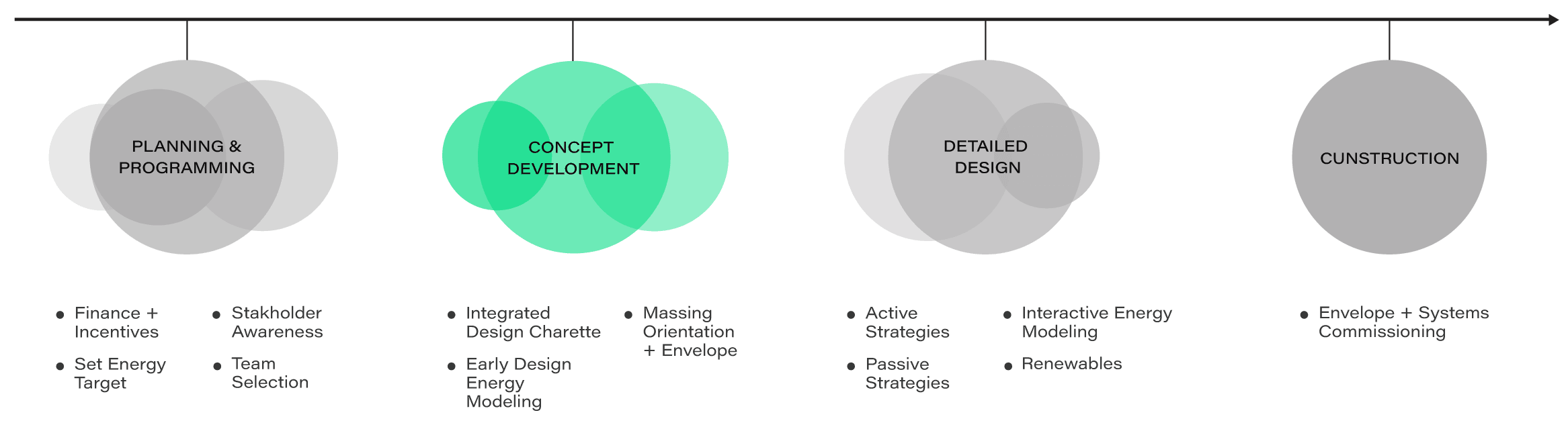
Building design at various stages and the role of simulation at the early stage
Our Philosophy on Energy Analysis for Architects
Considering the impact of decisions made during the early stages on the success of the final design solution, it is important to have an energy assessment from the earlier stages of decision-making, as suggested by the famous MacLeamy curve.
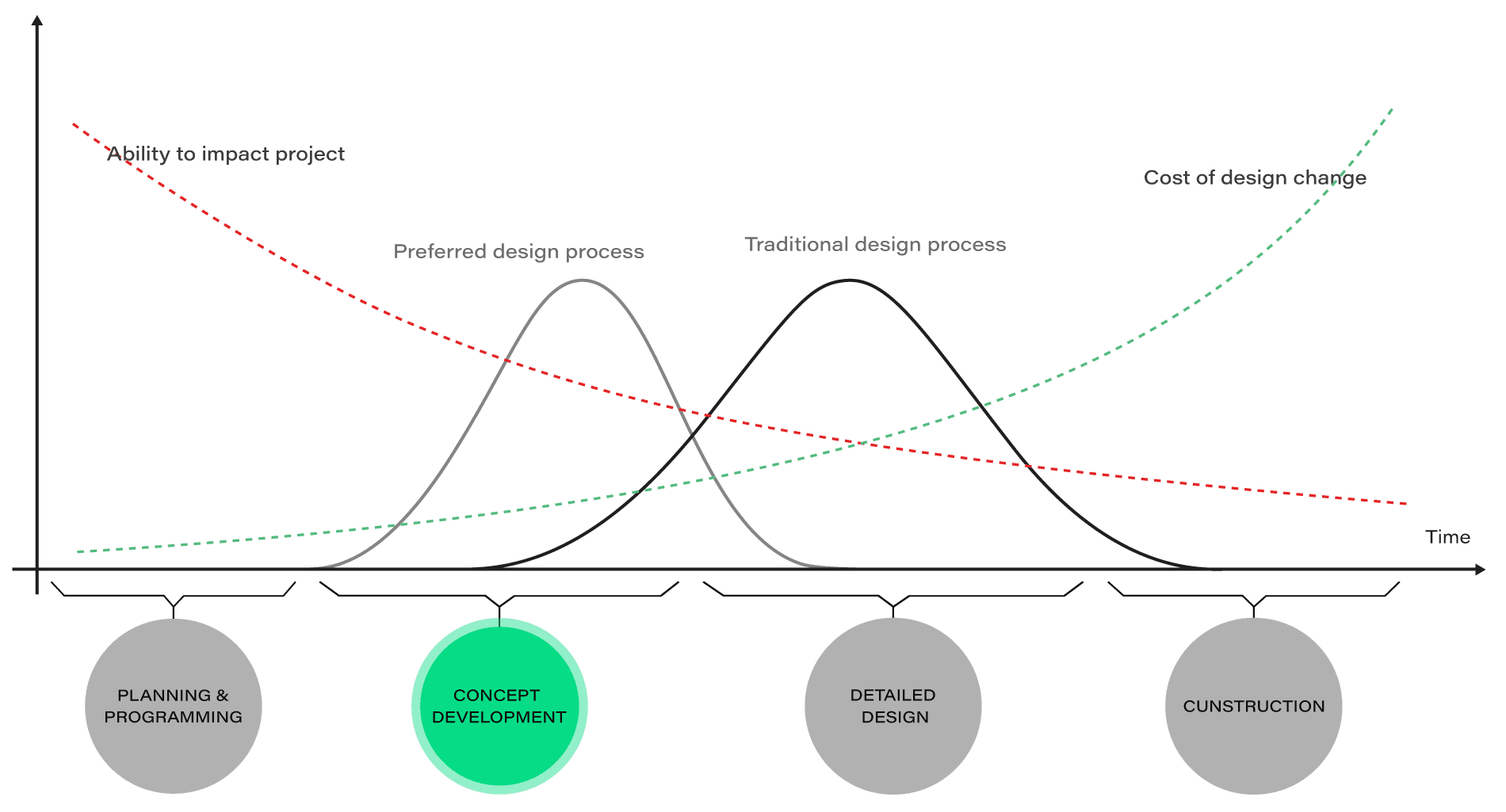
McLeamy Curve illustrates the notion that the further a project team is through the design process, the greater the cost of design changes. An integrative process shifts design efforts toward the front end of a project timeline- where there is maximum flexibility to make high-impact design decisions and interdisciplinary efforts are coordinated much sooner versus a more traditional design process.
In the conceptual and early phases of design development, when many design parameters are yet to be decided, it is advised to adhere to Occam’s razor or Einstein’s principle, which implies that a model should be as simple as possible but not simpler. The aim should be to keep the model as simple as possible to meet the objective of the simulation study. Thus, for the calculation of the energy and heat transfer in buildings at the early design stage, we apply reduced-order models that provide information with fewer inputs by implementing hourly normative equations. The main goal with such models is not necessarily the accurate prediction of future as-built performance, but an accurate comparison of the normative performance of design alternatives, i.e. an ordinal ranking of options. It also enables rapid, if course, investigation of the factors that impact performance.
Our Platform ArenPro
Based on this philosophy, we felt the need to develop a robust platform for simple building energy assessment that offers architects multiple approaches depending on their needs during various stages of building design. We take extensive knowledge and expertise from our team of PhDs in the domains of energy analysis, computational design, programming, and data science to provide the following energy analysis approaches:
- Simple hourly energy analysis tool for a design alternative
- A parametric study of various design alternatives using full-factorial
- Advanced optimization techniques with post-processing of the results
- Energy-driven generative design
- An inverse modeling approach for energy-informed design decision
Path to Zero Net Energy/Carbon
ZNE at ArenPro
Although ArenPro is not directly aimed to be applied for detailed zero net energy calculation, we suggest architects use the tool to evaluate the potential and paths to make their building close to zero net energy and carbon.
A zero net energy (ZNE) building is an energy-efficient building that produces as much energy as it consumes over the course of a year, usually by incorporating solar PV on-site. There are many different definitions of ZNE, all refer to operational energy use only. Achieving net zero is about reducing energy demand, and offsetting the last resort for residual emissions. Every project and site is different. Taking buildings on a journey towards net zero energy or carbon requires a sequential approach to ensure that critical decisions are made at the appropriate point in the design process.
As seen in the diagram below and applied in the parametric study approach, we offer the sequential approach necessary to arrive at a lasting net zero destination. To reduce energy use and operational carbon emissions, it is essential to:
- Passive Design: Begin with a strong passive design and minimize the need for active systems.
- Active System: Efficient systems are combined with operational tools to allow users and operators to choose how they will maintain net zero performance.
- Offsetting: Add in renewables and grid decarbonization and we are on a clear course toward net zero.
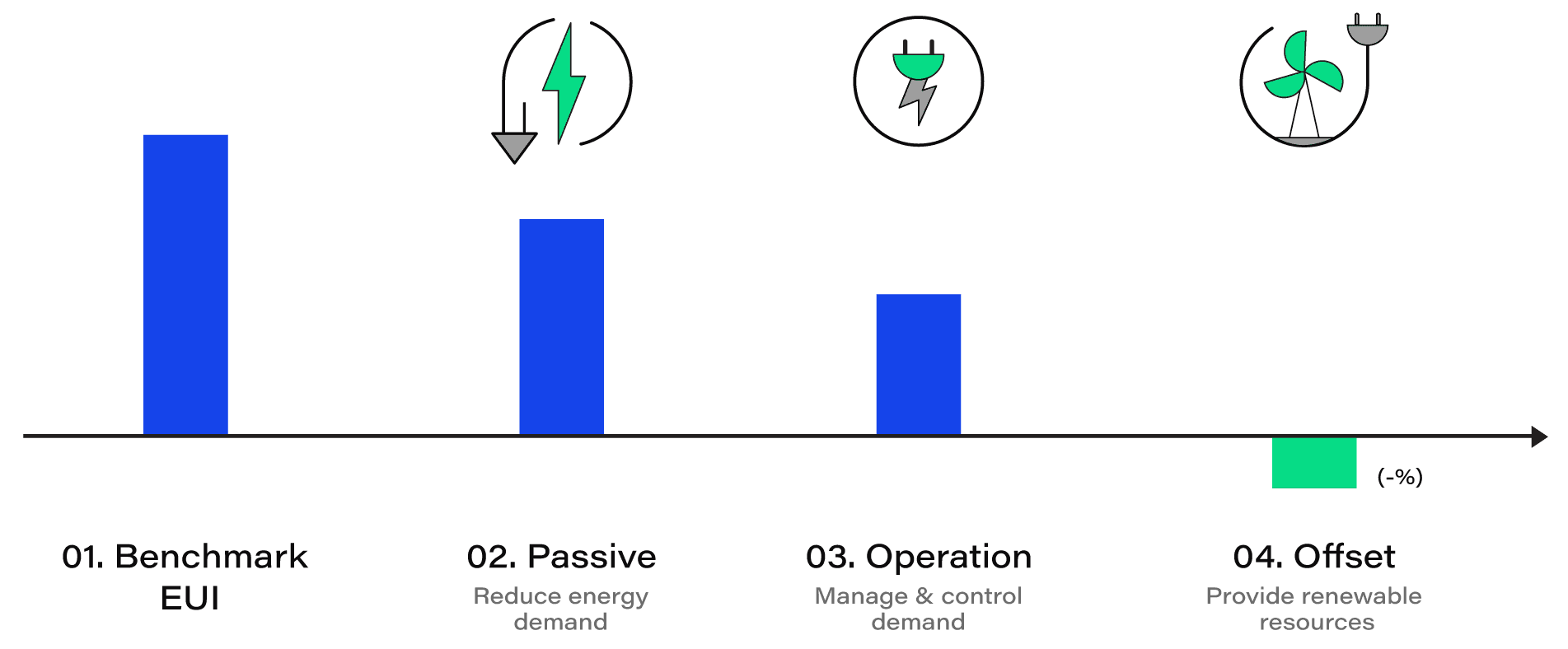
Sequencing Dacerbonization Interventions
Passive Design
Fundamental decisions made at the earliest stages of the design phase - including massing, floor-to-ceiling heights, and facade design - have significant carbon impacts through a building’s full lifecycle.
Goal:
- Reducing energy demand as much as possible
- Minimizing the need for active systems
- Minimizing the cost of the possible changes at the later stages
Design Parameters to Consider:
- Building Geometry Configuration
- Massing / Configuration
- Floor height
- Orientation
- Envelope
- Windows-to-wall ratio
- Assembly/Construction types
- Thermal Insulations
- Glazing SHGC
- Shading elements/ Passive solar gain
- Air-tightness
- Thermal bridge
- Others Solutions:
- Natural ventilation
- Thermal mass
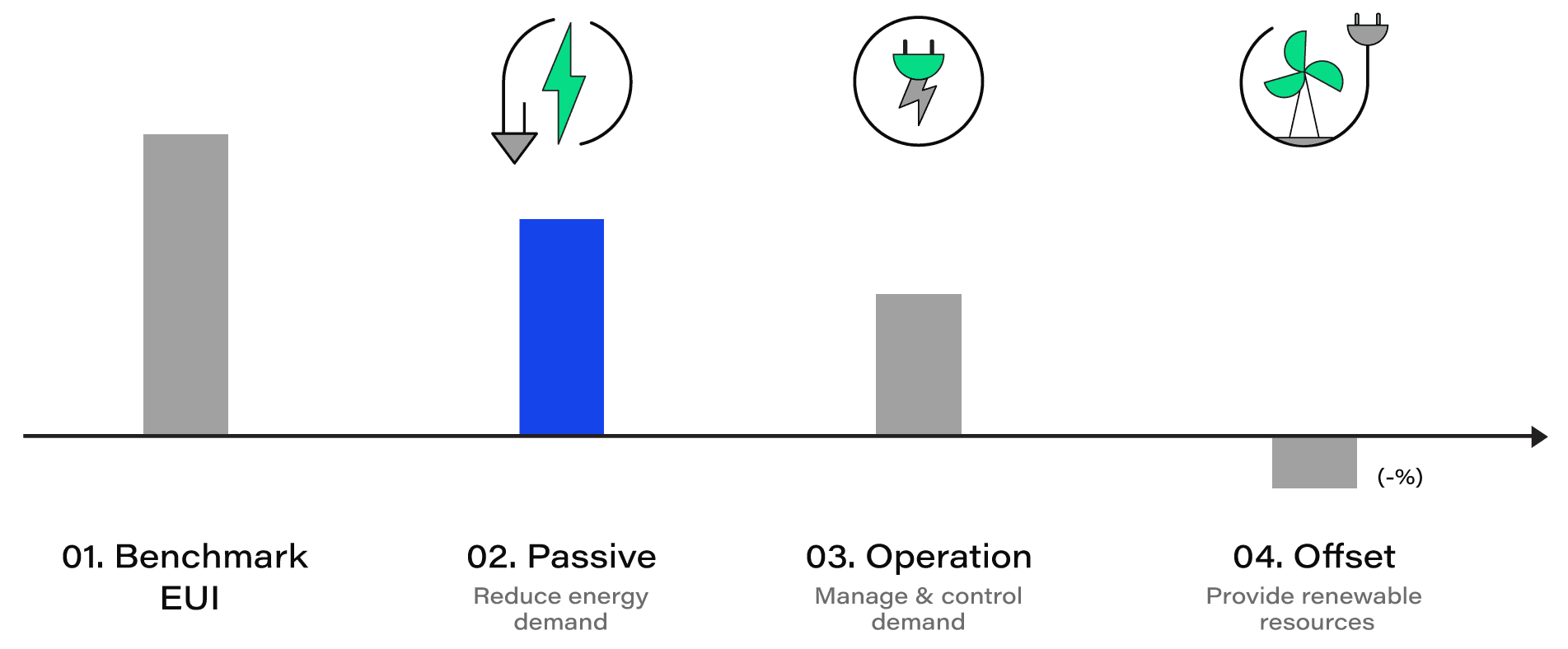
Active Systems
All systems - from heating and air conditioning to lighting - must be designed to be as efficient as possible with good control to maintain effective use. Minimizing operational carbon in this way is an important aspect of net zero design. Typically, supplying buildings with zero carbon energy means switching from an oil or gas supply to an electric system. Depending on building typology, it is likely that either heating or cooling will be the single biggest source of a building’s carbon use, and moving it to an electrical source will reduce building emissions as the electricity grid transitions away from fossil fuels and towards renewable and low carbon generation.
Reducing operational impacts is not just about how much energy is used overall, but when it’s used. Active demand management shifts energy demand away from peak periods when supply emissions are at their highest. It also allows for reduced infrastructure capacity, supporting building operators to make the best use of variable renewable generation.
Goal:
- Finding/designing low-energy HVAC
- Minimizing electric lighting and equipment
- Switching from oil/gas supply to electric system (reduce gas-fired heating)
- Lowering peak loads to reduce installation costs and improve efficiency
- Providing active demand management (shifting energy demand away from peak periods)
- Enabling Smart control to minimize day-to-day energy demand
Paremeters to Consider:
- HVAC
- HVAC types
- Efficient components (HRV, economizer, ,,)
- Mixed mode ventilation (natural, evaporative, ,,)
- LPD/EPD
- Energy-efficient lighting
- Energy-efficient equipment
- Control and Monitoring System
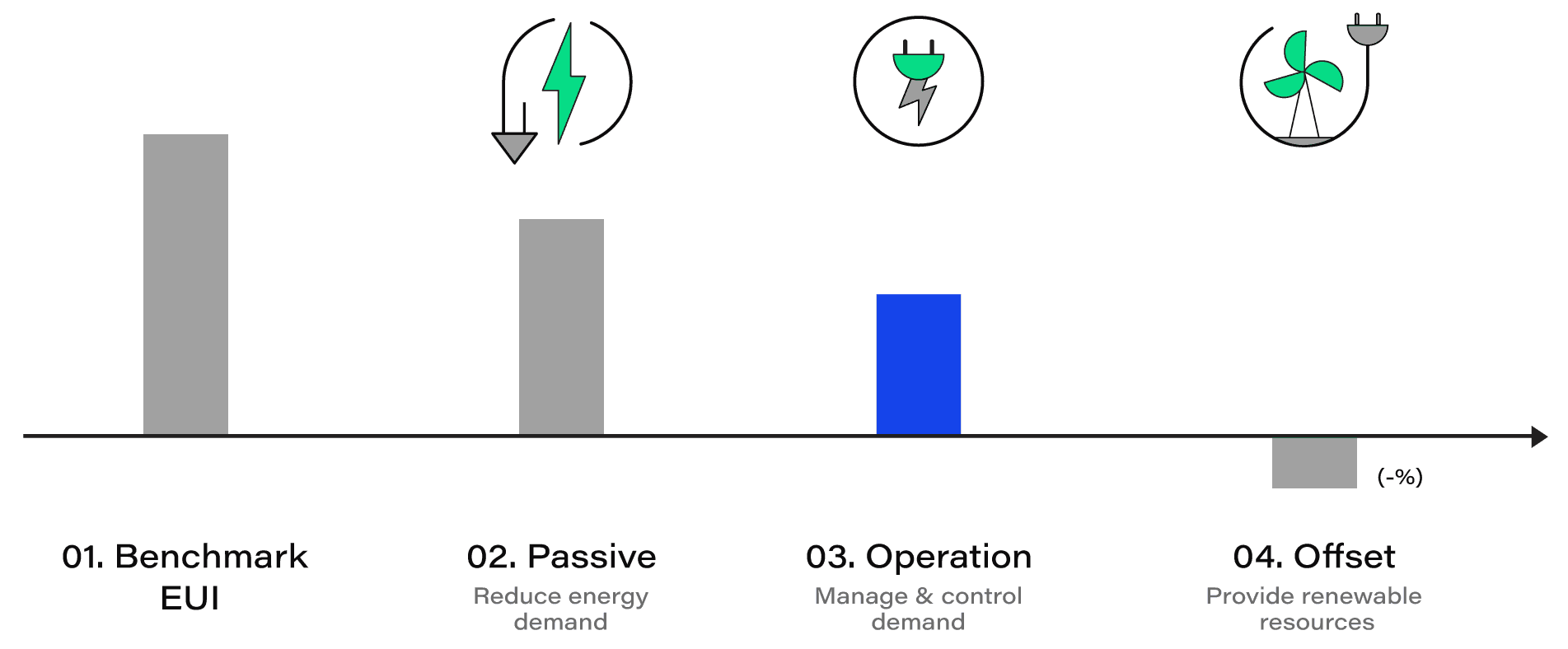
Offsetting
Where practicable, onsite renewable generation, such as solar photovoltaic (PV) panels, should be explored. However, a lifecycle cost and carbon appraisal should be conducted to determine the feasibility of any onsite renewable generation. Not all sites or buildings can generate enough renewable energy to make installation worthwhile.
Goal:
- Maximizing renewable generation
- Reducing infrastructure capacity
- Utilizing the green resources
Parameters to Consider:
- Onsite Renewables
- PV, BIPV, wind, geothermal,,,
- Purchasing Possibilities
- Renewable power purchase
- Green lease
- Resources | Grid Level
- District heating/cooling systems
- Right sizing plant
- district energy networks to enable energy sharing
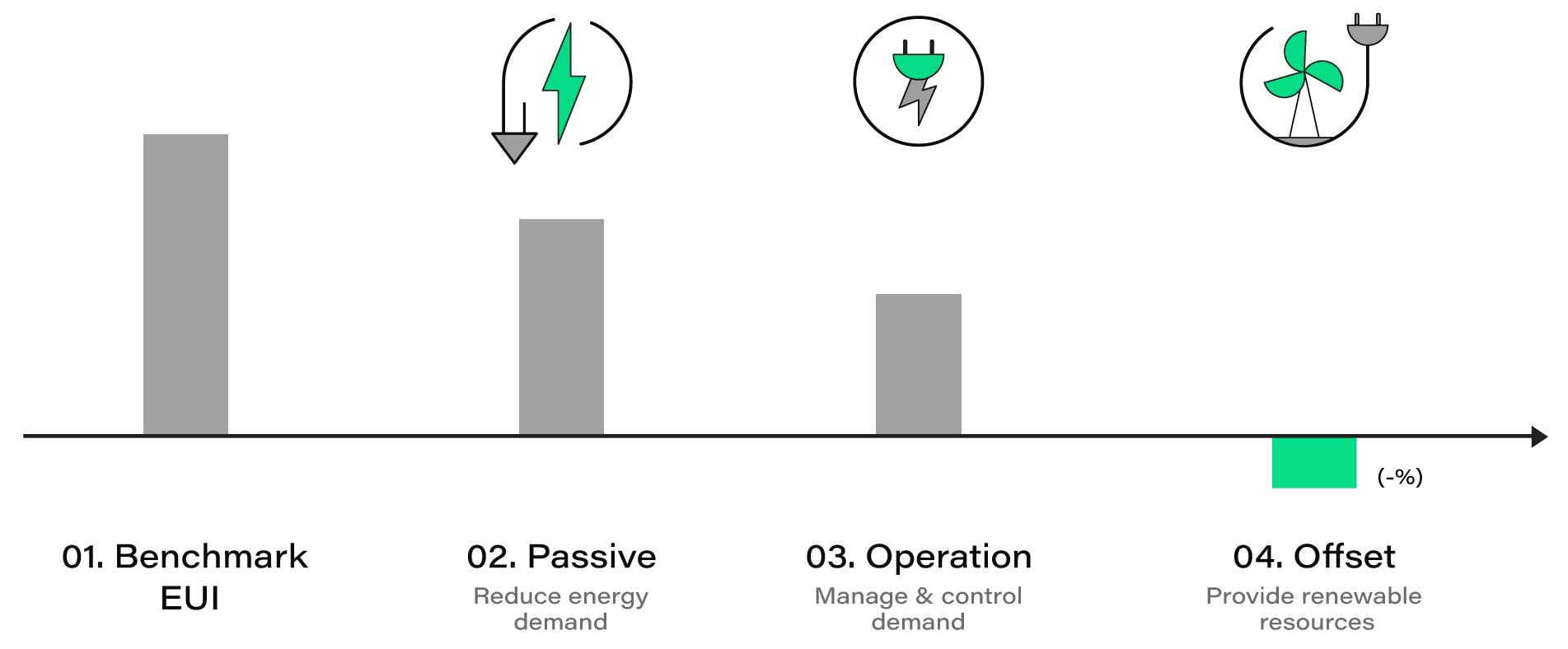
ArenPro Methods for Energy-Informed Design Decisions
Single Run-Baseline
A single-run analysis is a simple process of calculating the energy consumption for one set of inputs using hourly normative equations. We consider the initial user inputs as the "Baseline" that is going to be compared to other input scenarios in the following steps using a parametric study.
Parametric Study (Full-Factorial)
A parametric analysis studies the influence of different geometric or physical parameters, or both, on the problem's solution. Parametric analysis at ArenPro is an essential tool for design exploration, for instance, to examine the influence of floor height or envelope insulation on the energy consumption in a building. The parametric study enables you to perform trade-off studies and explore ‘what-if’ scenarios. You can examine how your building behaves as you vary one or more input parameters.
We make it simple to analyze model behavior in this way. All you have to do is to set a list of alternative values to each input parameter and run the analysis. We use full factorial enabling you to consider all combinations of parameters. The results are shown in a PCP (Parallel Coordinate Plot), which displays a graph of how it varies for each combination of the input parameter
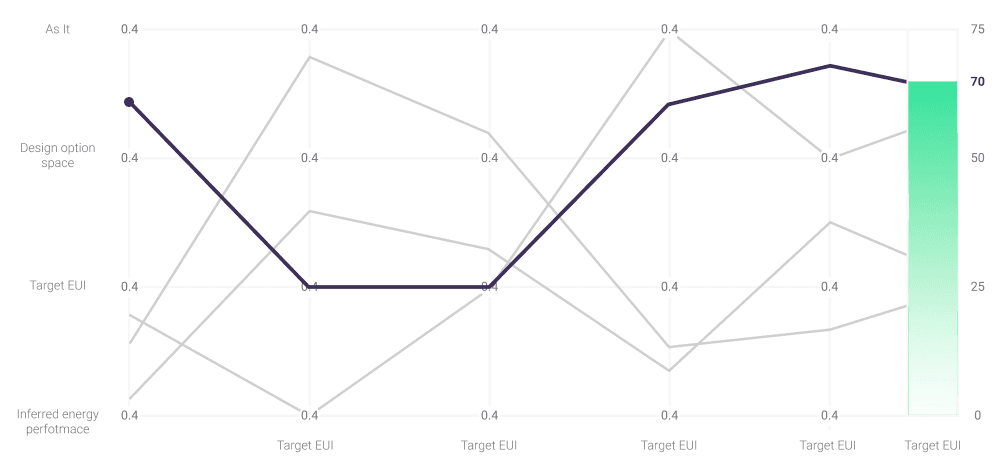
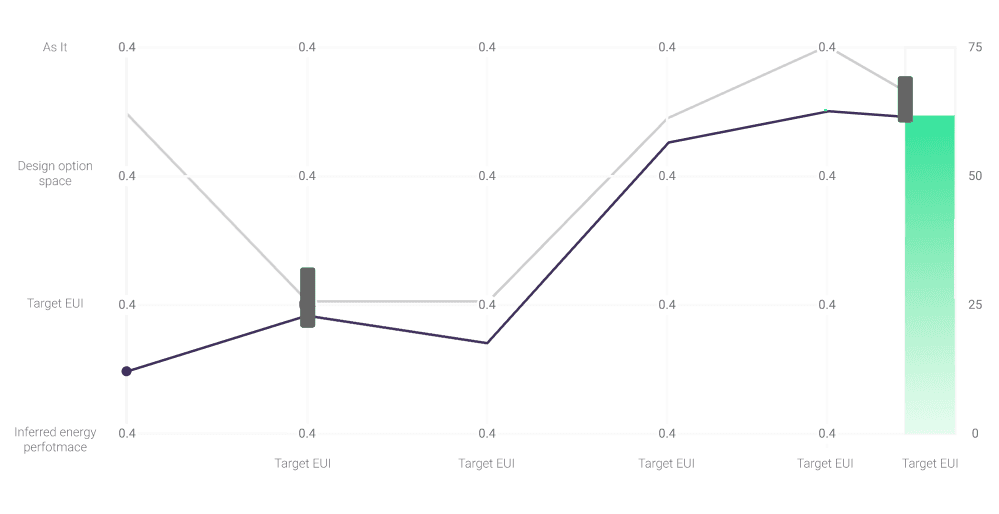
Optimization (Random Sampling)
Optimization is intended to produce an optimal solution or an optimal compromise between competing parameters. Unlike parametric analysis which requires an initial design for examination, optimization offers engineers a blank slate to explore the design space and create optimal designs based on the constraints defined by the user. The optimization approach at ArenPro is based on deterministic algorithms, which do not consider any uncertainties.
Generative Design
Generative design (GD) is a process of defining high-level goals and constraints and using the power of computation to automatically explore wide design space and identify the best design options. Think of generative design software as an assistant that helps with creating, testing, and evaluating options. ArenPro is utilizing algorithmic problem-solving technology to explore design alternatives, identify improved outcomes in terms of energy performance, and make data-informed decisions faster than ever before.
The generative design allows for a more integrated workflow between humans and computers. This workflow involves the following stages:
- Generate: This is the stage when design options are created or generated by the system, using algorithms and parameters specified by the designer.
- Analyze: The designs generated in the previous step are now measured or analyzed on how well they achieve the goals defined by the designer.
- Rank: Based on the analysis results, design options are ordered or ranked.
- Evolve: The process uses the ranking of the design options to figure out in which direction designs should be further developed or evolved.
- Explore: The designer compares and explores the generated designs, inspecting the results based on their original criteria.
- Integrate: After choosing a favorite design option, the designer uses or integrates this design into the wider project or design work.
Inverse Modeling
In contrast to the conventional forward energy analysis approaches in which design parameters are input and considered to be known- and energy performance is output, in the inverse modeling approach, the objective energy performance is the input and the design parameters-which are unknown- are the output. Inverse modeling is used in ArenPro to provide estimates of the most important inputs from the preferred energy use outcome. This is an innovative approach applied to the ArenPro platform for the first time.
ArenPro uses linear inverse modeling (LIM) for solving the inverse equation. In a LIM, energy performance is described as a linear function of design parameters. The following figure graphs the methodology that generates the design solution. It starts from the design options space, which consists of the design parameters, x, with possible upper and lower limits, represented probabilistically. The initial uniform distributions show all feasible values for the design parameters based on the project requirements and constraints. Using the linear function f (x) that provides a relationship between design parameters and energy performance, the model derives the response space as a distribution of the energy performance indicator. It then selects a subset of the response space corresponding to the objective energy performance and uses it in an inverse algorithm to estimate distributions of the initial design variables, as solution space.